Plenty of people believe the old bathroom mirror is brutally honest, but they might be shocked by how much more a neural network notices. In 2024 the global beauty-tech market was already worth about USD 66 billion, and analysts expect it to top USD 172 billion by 2030. Behind that curve sits a simple idea: better data makes better routines. For decades dermatologists relied on visual inspections and a patient’s memory. Today a smartphone camera, spectral scanner, or at-home genomic kit can capture thousands of data points in seconds. Give those inputs to machine-learning models, and the result is guidance that feels almost psychic. I still remember the first time a vision algorithm flagged dehydration in my cheek before I felt it – reality has a way of outpacing science fiction!
The surge of artificial intelligence in beauty did not start with robots handing out lipstick. It began quietly with ingredient databases, predictive toxicology, and color-matching engines tucked behind e-commerce sites. When consumers clicked “find my shade,” a convolutional neural network chugged through millions of labeled images to return a near-perfect match. That small success rewired expectations. If software can handle makeup, why not the trickier problems of barrier disruption, hormonal acne, or photoaging? Investors noticed, and start-ups sprinted to patent algorithms that promise clinically significant improvements delivered through personalized routines. The result is a market moving faster than regulatory frameworks and far faster than shelf-life studies were designed to track.
Skeptics worry that AI will oversell its abilities. They are right to ask hard questions. Yet the direction of travel is unmistakable. Every major beauty conglomerate now operates an AI lab or has acquired a tech start-up to accelerate customization. Even independent brands leverage off-the-shelf APIs to scan selfies and recommend serums tuned to local humidity. A race is on: who can convert raw consumer data into the kind of intimate, helpful advice historically reserved for one-on-one dermatology visits? Once that advice is accurate and instant, the notion of “mass” product lines begins to wobble.
From Selfies to Molecular Maps
Modern computer vision has reached a point where a phone image offers more than a dermatologist’s quick look under clinic lighting. Vision models trained on datasets annotated by experts can classify redness, wrinkle depth, pore visibility, and pigment clusters across multiple Fitzpatrick skin types. They translate pixel variations most humans ignore into quantifiable metrics – exactly the language needed for precise formulation tweaks. Some systems, such as Samsung’s AI Beauty Mirror presented at CES, even simulate future aging under different lifestyle scenarios (Vogue Business). The shift from subjective “looks dry” to objective “stratum corneum hydration dropped eight percent overnight” lays the foundation for hyper-personal routines.
Sensor platforms push that boundary further. Portable Raman spectrometers read molecular vibrations on skin, revealing lipid balance or sunscreen residue. AI models interpret those spectra in real time, suggesting an emollient booster or a fresh coat of SPF. Meanwhile, microbiome sequencing costs continue to fall. Brands now mail swab kits, feed the DNA reads into machine-learning pipelines, and return probiotic-friendly cleanser recommendations within days. The skin, once mysterious, is turning into a dataset that can be sliced, graphed, and optimized.
None of this works without enormous ingredient libraries. That is why cosmetic chemists partner with data scientists to annotate raw materials – chemical structure, polarity, irritation index, sourcing footprint, and more. Transformer models search these tables the way language models parse sentences, except the output is a shortlist of actives predicted to calm inflammation without destabilizing a formula’s viscosity. When that shortlist connects to supply-chain APIs, brands can check real-time availability or carbon footprint before committing to a batch. It is formulation as a living document, updated the moment new data arrives.
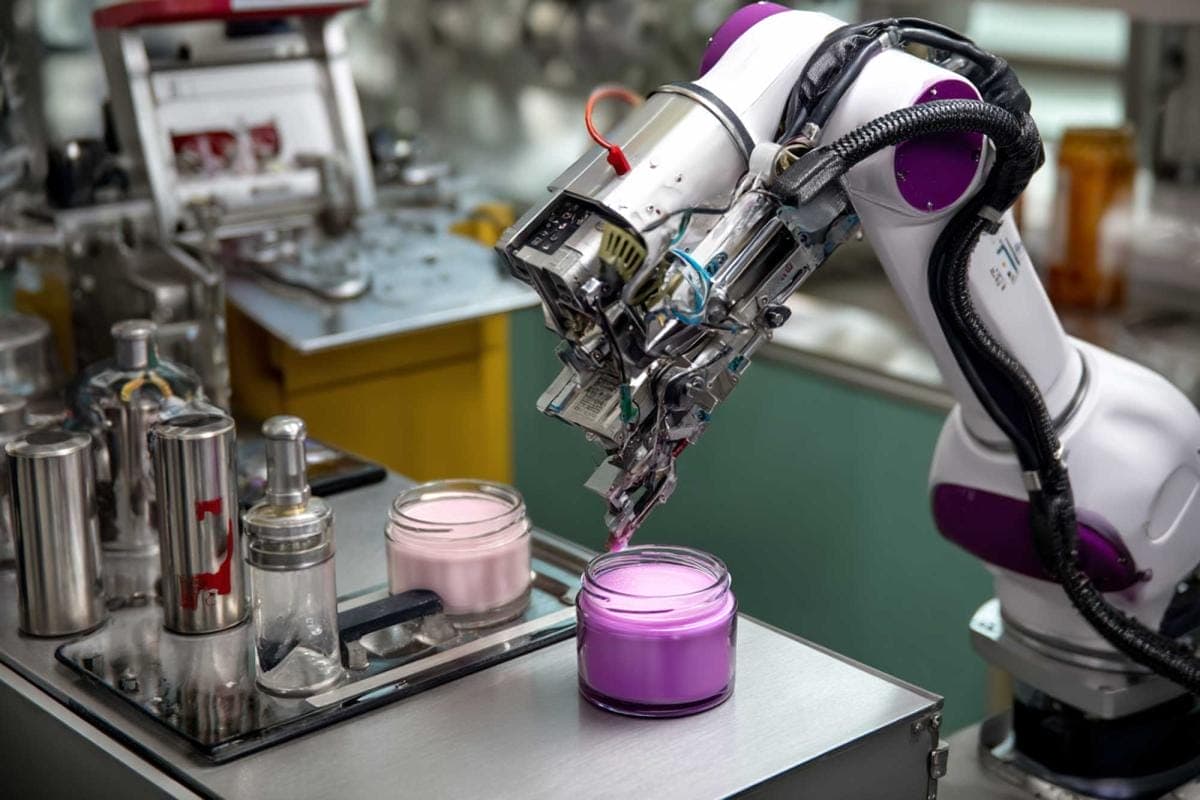
The Engine Inside Personalized Formulas
Once data capture is robust, personalization hinges on matching skin profiles to the right blend of ingredients at the right dose. Traditional rules of thumb – “2 percent niacinamide suits most users” – give way to gradient-boosting models that forecast efficacy curves for age, environment, diet, and prescription history. In practice that means two friends ordering anti-aging cream on the same website may receive jars with different peptide ratios, even if the label looks identical.
Manufacturing adapts through micro-batch systems reminiscent of espresso machines. A foundation kiosk in Seoul already mixes 205 base shades on demand, guided by AI color analysis of a client’s face. Sales data show a notable uptick in repeat purchases and shade accuracy satisfaction (Reuters). Skincare is moving in the same direction. Cartridge systems store actives in concentrated pods, and robotic nozzles blend them into a neutral base only when an order arrives. Such agility slashes preservative load – fresh cream does not need a three-year shelf life – and reduces product waste because inventory sits in bulk components, not finished units.
Artificial intelligence also refines dosage over time. Feedback loops ingest user-reported outcomes, app-captured selfies, or smart-mirror scans. Regression models spot that a customer’s transepidermal water loss fell after a Week 3 tweak but melasma clusters remained unchanged. The system then nudges up tranexamic acid while keeping ceramide levels steady – iterations too small for manual formulating but meaningful at scale. This continuous adjustment blurs the line between product and service. You are no longer buying a cream; you are subscribing to an algorithm’s evolving recipe.
Retail Rethinks the Beauty Counter
Brick-and-mortar stores feel the ripple effect. Beauty advisors once relied on product training and color wheels; now they wield tablet apps tied to the cloud. A customer’s purchase history, lifestyle questionnaire, and last skin scan appear instantly. The advisor’s job transforms from recommending stock keeping units to curating bespoke blends, occasionally printing a fresh mask in a back-of-store 3D bioprinter loaded with botanical hydrogels.
For retailers the upside is loyalty. Consumers who receive a serum “made for me” are statistically less likely to swap brands, even if a competitor offers a similar promise. Ulta Beauty, LVMH, and other giants have invested in start-ups that specialize in AI-driven personalization kiosks (Vogue Business). They treat the technology as both a revenue engine and a data mine. Each scan yields demographic insights, regional trends, and early warning signals for inventory planning. When aggregated at scale, those metrics influence everything from store layout to marketing language.
E-commerce mirrors this trend through virtual try-on and live recommendation engines. Instead of scrolling endless listings, shoppers upload a selfie, flag skin concerns, and receive a ranked regimen with auto-refill scheduling. Some platforms embed conversational chatbots fine-tuned on dermatology research. Ask a question about glycolic acid at 2 a.m., and the AI answers within seconds, citing peer-reviewed studies and linking to patch-test kits. The experience feels less like shopping and more like having a personal derm sitting in your pocket.
Safety, Ethics, and Trust in the Age of Smart Skin
The promise of AI is seductive, but it cannot exist without trust. Skincare touches health, and that makes data sensitivity paramount. High-resolution facial images can identify individuals; genomic reads reveal ancestry and disease risk. Brands must encrypt, anonymize, and obtain explicit consent for every data point stored. Regulations such as the European Union’s AI Act already classify facial analysis for medical purposes as high risk, demanding stringent testing and human oversight.
Bias is another hurdle. Training datasets that underrepresent deeper skin tones will misclassify hyperpigmentation or underestimate dehydration in those users. The solution lies in painstaking data curation, transparent algorithmic audits, and partnerships with diverse dermatology cohorts. Brands that invest early will avoid public relations crises and, more importantly, provide accurate care to every customer.
Ingredient safety modeling introduces a different ethical layer. Predictive toxicology tools flag molecules likely to irritate, but false negatives can slip through when chemical families are underrepresented in training data. Human chemists remain indispensable gatekeepers, reviewing AI suggestions before any pilot batch hits a customer’s doorstep. In effect, the future is not machines replacing formulators; it is machines handling search while humans apply judgment.
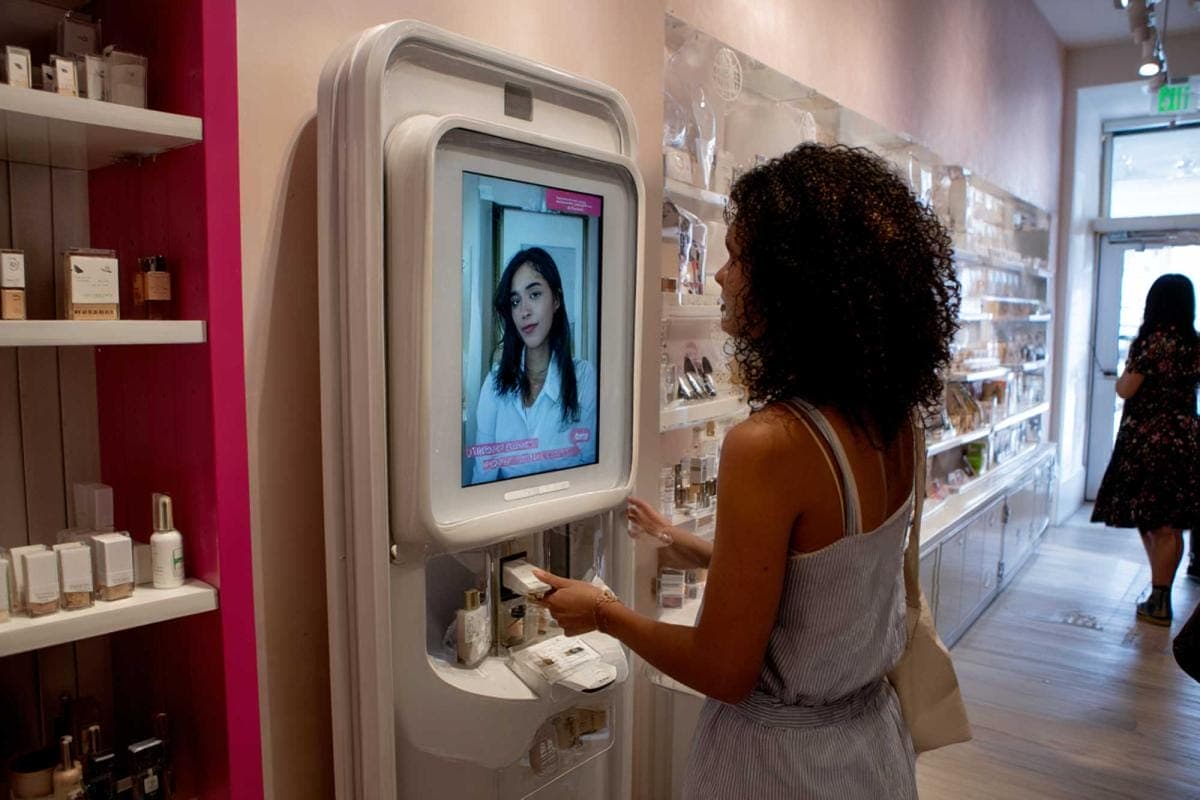
What Happens Next
Five trends point to the horizon. First, multimodal AI will merge skin scans with lifestyle inputs – sleep trackers, pollution sensors, even oral microbiome readings – to generate holistic routines that adjust in real time. Second, longitudinal cohort studies fed into federated learning frameworks will let brands improve algorithms without moving raw data off users’ devices, easing privacy concerns. Third, 3D printing will extend beyond sheet masks to create layered patches that release actives at controlled intervals, triggered by pH shifts detected through micro-sensors.
Fourth, sustainability metrics will embed directly into recommendation engines. Consumers may choose a serum not only for efficacy but for its predicted CO₂ footprint based on ingredient sourcing, transportation distance, and recycling rates. AI optimizes logistics, filling local warehouses with the precise split of actives needed for next week’s orders, cutting both waste and emissions.
The fifth trend involves emotional analytics. Early research suggests facial micro-expressions can betray discomfort before a user consciously reports stinging or itching. AI that detects such cues during a patch-test video call could pause a routine, dispatch a soothing product, or flag possible allergies for a clinician. The boundary between skincare and telehealth blurs, offering preventive intervention rather than reactive correction.
FAQ
Why does AI outperform manual skin assessment?
Traditional evaluations rely heavily on visual estimates and patient recall. AI integrates high-resolution imagery, spectral data, and even genomic information to uncover patterns invisible to the naked eye. By comparing your profile against millions of annotated cases, algorithms can isolate specific drivers of dryness or sensitivity with statistical confidence. That breadth of data makes the analysis both faster and more granular, enabling targeted interventions that a single clinician could miss across a busy day’s appointments. The technology does not replace professional expertise; it augments it by flagging subtle cues and providing quantitative baselines users and doctors can track over time.
How accurate are personalized AI formulations?
Accuracy depends on model training, input quality, and manufacturing precision. Leading platforms validate recommendations through blinded clinical trials, comparing personalized blends against placebo or generic formulations. Results often show significant improvements in hydration, wrinkle depth, or redness reduction within eight to twelve weeks. Continuous feedback loops refine these gains. If weekly selfies indicate uneven tone persists, the algorithm incrementally boosts actives like niacinamide while monitoring potential irritation. The iterative cycle ensures the product evolves alongside your skin’s changing needs, delivering a level of adaptability that static shelf products cannot match.
What about data privacy?
Facial images and genomic reads qualify as sensitive personal data in many jurisdictions. Responsible companies therefore encrypt files at rest and in transit, employ differential privacy techniques, and minimize retention periods. Some adopt federated learning, where models train locally on your device, sharing only anonymized gradients with a central server. Regulatory bodies such as the EU and several U.S. states mandate transparent consent forms that specify data usage, storage duration, and third-party access. Consumers should look for clear privacy policies, independent security audits, and the ability to delete their datasets with a single request. Trust remains non-negotiable; without it, personalization collapses.
Will AI make beauty professionals obsolete?
On the contrary, it expands their toolkit. Estheticians and dermatologists gain dashboards full of objective metrics, freeing them to focus on nuanced interpretation and patient education. Retail associates shift from memorizing product catalogs to guiding customers through biometric scans and explaining ingredient science in plain language. Chemists accelerate R&D by screening virtual molecule libraries in silico before ordering physical samples. In each case, AI handles repetitive computation while humans supply empathy, creativity, and ethical oversight – the qualities automation still struggles to replicate.